Finding Faults in Classification Models through Error Isolation
Program:
Applied Mathematics and Statistics
Project Description:
In the rapidly evolving field of data-driven decision-making, the accuracy and reliability of predictive models are important. In particular, classification models (such as linear or nonlinear classifiers), enjoy widespread application across various domains. This project addresses the critical gap in error identification and isolation within classification models, which, despite their popularity, lack specialized methods for diagnosing misclassifications. By integrating Bayesian inference principles and adopting Monte Carlo methods for complex integral computations, we propose a novel approach to enhance classification performance. Our methodology demonstrates significant promise in isolating incorrectly specified (faulty) parameters in 2D logistic regression examples, paving the way for future applications in high-dimensional models and real-world scenarios. This study not only contributes to the refinement of classification analysis but also underscores the importance of robust error detection mechanisms in the broader context of engineering and artificial intelligence.
Team Members
-
[foreach 357]
[if 397 not_equal=””][/if 397][395]
[/foreach 357]
Project Mentors, Sponsors, and Partners
James Spall
Course Faculty
-
[foreach 429]
[if 433 not_equal=””][/if 433][431]
[/foreach 429]
Project Links
Additional Project Information
Project Photo
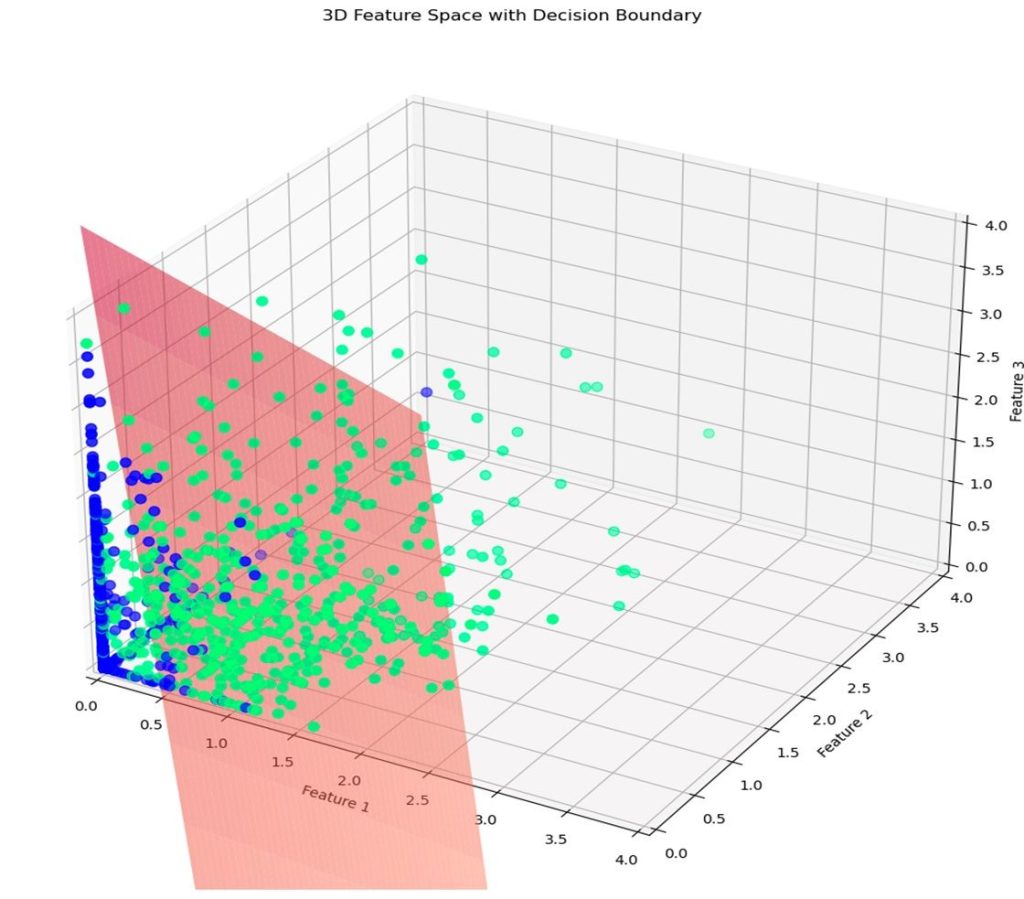
Project Photo Caption:
3D Feature Space with Boundary